Understanding the Role of Training Data for Self-Driving Cars
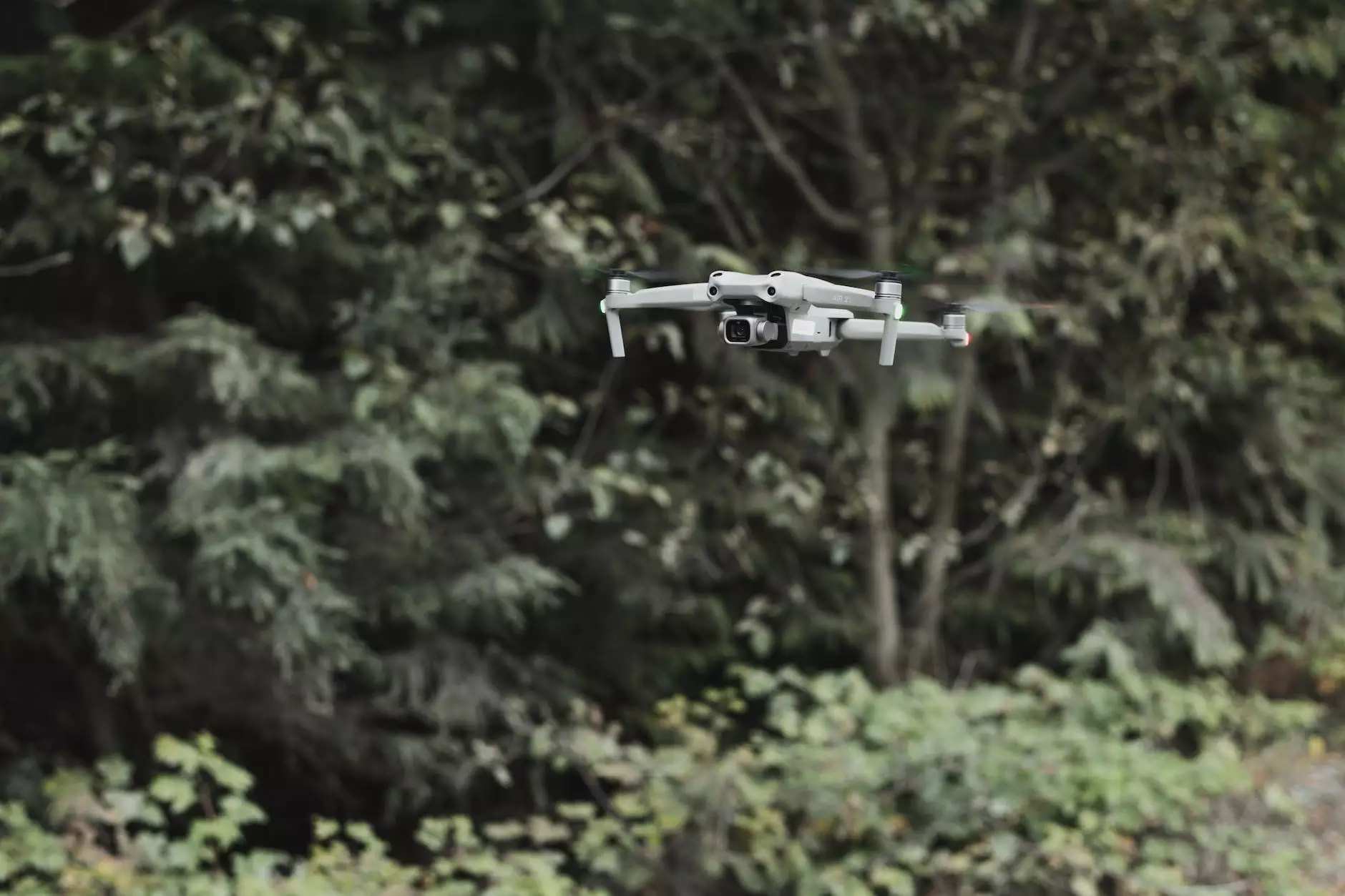
The advancement of autonomous vehicles is one of the most transformative developments in the field of technology. At the heart of this transformation lies the essential concept of training data for self-driving cars. This article delves deep into how effective training data fuels machine learning algorithms, propels software development, and continuously shapes the future of transportation.
What is Self-Driving Technology?
Self-driving technology, often referred to as autonomous driving, mimics human driving capabilities through the utilization of various sensors, software, and deep learning frameworks. The technology relies heavily on artificial intelligence (AI) to interpret input from a myriad of data sources and to execute appropriate driving actions.
Understanding Training Data
Training data is the foundational element that enables machines to learn from patterns. In the context of self-driving cars, training data consists of vast amounts of information collected during various driving conditions to enhance the accuracy and safety of autonomous systems. The presence of robust and high-quality training data is vital for robust AI performance.
The Components of Training Data
- Sensor Data: Vehicles equipped with cameras, LiDAR, radar, and ultrasonic sensors generate extensive streams of data regarding their surroundings.
- Driving Scenarios: From day to night, clear weather to harsh storms, training data needs to encapsulate diverse driving conditions.
- Annotated Data: Object detection, semantic segmentation, and lane marking require precise annotations for effective machine learning.
How Training Data Enhances Machine Learning
Machine learning thrives on data quality. For self-driving cars, having a comprehensive dataset ensures that the AI can accurately recognize objects, predict scenarios, and respond appropriately. This section discusses how training data impacts the learning process of self-driving technology:
1. Improved Object Recognition
One of the primary functionalities of self-driving cars is their ability to recognize obstacles, pedestrians, and other vehicles. High-quality training data allows models to learn from a variety of images and scenarios, improving their accuracy in object classification and recognition.
2. Better Decision Making
Training data helps AI algorithms make smart decisions based on past experiences. A self-driving system can learn to navigate complex situations — such as merging onto highways or stopping at traffic signals — by training on data that illustrates numerous decision-making scenarios.
3. Adaptability to Real-World Conditions
A critical aspect of autonomous driving is the ability to adapt to unpredictable environments. By utilizing extensive training data that encompasses various conditions, self-driving cars can enhance their resilience and performance across a range of real-world situations.
The Importance of Quality in Training Data
The emphasis on quality cannot be overstated when it comes to training data for self-driving cars. Poor-quality data can lead to inefficient learning models, fostering safety risks and operational failures. Here are key aspects of ensuring quality:
- Diverse Dataset: Incorporating data from diverse geographical locations, weather conditions, and urban vs. rural settings enhances the learning experience.
- Consistent Labeling: High accuracy in data annotation, including precise boundaries for objects in the visual input, is critical for predictive models.
- Continuous Updates: As driving regulations evolve and new technologies emerge, regularly updating training datasets is essential for maintaining performance and safety standards.
Implementing Effective Data Collection Strategies
For companies engaged in the development of self-driving technology, implementing a robust data collection strategy is essential. Here are some strategies to enhance data collection:
1. Utilizing Real-World Testing
Conducting extensive on-road testing provides authentic training data collected from real driving conditions. This method allows the technology to observe and learn from genuine interactions on the road.
2. Synthetic Data Generation
To supplement real-world data, companies often utilize simulations and synthetic data generation. These technologies create diverse virtual scenarios that challenge the AI and generate data that may be difficult to collect in reality, thereby enhancing its learning capabilities.
3. Collaborative Data Sharing
Collaboration between different companies working on self-driving technologies can facilitate data sharing agreements. By pooling high-quality data, companies can improve their machine learning models significantly.
Impact on Software Development
The intersection between training data and software development is a pivotal element in the advancement of autonomous vehicles. Investing in software capable of processing and utilizing large datasets efficiently is crucial. Companies like Keymakr, specializing in software development, are leading in creating tailored solutions for handling and analyzing this data.
1. Agile Methodologies
The iterative nature of agile methodologies allows software development teams to refine models and algorithms rapidly in response to performance metrics. By utilizing quality training data effectively, teams can enhance software functionalities in shorter cycles.
2. Enhanced Testing Protocols
Effective use of training data allows for comprehensive testing scenarios, reducing risks associated with software errors. Advanced testing frameworks can simulate the impact of diverse driving conditions, ensuring that software behaves optimally in real-world situations.
Challenges in Training Data for Self-Driving Cars
While the use of training data is crucial, there are inherent challenges that must be addressed:
1. Data Privacy Concerns
Collecting and utilizing data from real-world drivers poses privacy risks. Companies must ensure compliance with data protection regulations and ethical standards when gathering driving data.
2. Data Imbalance
A potential issue in training datasets is data imbalance, where certain scenarios may be overrepresented while others are underrepresented. This imbalance can lead to poor performance in less frequent situations.
Future Trends in Training Data for Self-Driving Cars
As we look toward the future of autonomous driving, several trends are expected to shape the paradigm of training data:
1. Advances in Machine Learning Techniques
The use of advanced machine learning approaches, such as deep learning, reinforcement learning, and anomaly detection, will enhance the efficacy of utilizing training data, leading to smarter and safer autonomous systems.
2. Integrated Data Ecosystems
Ecosystem integrations, where various data sources interact seamlessly, will provide a more comprehensive dataset that can be utilized for training. This interconnectedness will foster improved decision-making and safety outcomes.
3. Real-Time Data Processing
Future advancements may focus more on real-time data processing capabilities, allowing self-driving cars to adapt dynamically to their environments by processing data as it becomes available rather than relying solely on pre-collected data.
Conclusion
The journey toward fully autonomous vehicles is heavily reliant on the quality and efficacy of training data for self-driving cars. As the demand for safer transportation solutions increases, the importance of investing in comprehensive data strategies and software development efforts cannot be overstated. By embracing innovation and developing high-quality training datasets, companies like Keymakr are positioning themselves at the forefront of this exciting technological evolution, paving the way for a new era of transportation.
training data for self driving cars